Kategorie
Ebooki
-
Biznes i ekonomia
- Bitcoin
- Bizneswoman
- Coaching
- Controlling
- E-biznes
- Ekonomia
- Finanse
- Giełda i inwestycje
- Kompetencje osobiste
- Komputer w biurze
- Komunikacja i negocjacje
- Mała firma
- Marketing
- Motywacja
- Multimedialne szkolenia
- Nieruchomości
- Perswazja i NLP
- Podatki
- Polityka społeczna
- Poradniki
- Prezentacje
- Przywództwo
- Public Relation
- Raporty, analizy
- Sekret
- Social Media
- Sprzedaż
- Start-up
- Twoja kariera
- Zarządzanie
- Zarządzanie projektami
- Zasoby ludzkie (HR)
-
Dla dzieci
-
Dla młodzieży
-
Edukacja
-
Encyklopedie, słowniki
-
E-prasa
- Architektura i wnętrza
- BHP
- Biznes i Ekonomia
- Dom i ogród
- E-Biznes
- Ekonomia i finanse
- Finanse
- Finanse osobiste
- Firma
- Fotografia
- Informatyka
- Kadry i płace
- Komputery, Excel
- Księgowość
- Kultura i literatura
- Naukowe i akademickie
- Ochrona środowiska
- Opiniotwórcze
- Oświata
- Podatki
- Podróże
- Psychologia
- Religia
- Rolnictwo
- Rynek książki i prasy
- Transport i Spedycja
- Zdrowie i uroda
-
Historia
-
Informatyka
- Aplikacje biurowe
- Bazy danych
- Bioinformatyka
- Biznes IT
- CAD/CAM
- Digital Lifestyle
- DTP
- Elektronika
- Fotografia cyfrowa
- Grafika komputerowa
- Gry
- Hacking
- Hardware
- IT w ekonomii
- Pakiety naukowe
- Podręczniki szkolne
- Podstawy komputera
- Programowanie
- Programowanie mobilne
- Serwery internetowe
- Sieci komputerowe
- Start-up
- Systemy operacyjne
- Sztuczna inteligencja
- Technologia dla dzieci
- Webmasterstwo
-
Inne
-
Języki obce
-
Kultura i sztuka
-
Lektury szkolne
-
Literatura
- Antologie
- Ballada
- Biografie i autobiografie
- Dla dorosłych
- Dramat
- Dzienniki, pamiętniki, listy
- Epos, epopeja
- Esej
- Fantastyka i science-fiction
- Felietony
- Fikcja
- Humor, satyra
- Inne
- Klasyczna
- Kryminał
- Literatura faktu
- Literatura piękna
- Mity i legendy
- Nobliści
- Nowele
- Obyczajowa
- Okultyzm i magia
- Opowiadania
- Pamiętniki
- Podróże
- Poemat
- Poezja
- Polityka
- Popularnonaukowa
- Powieść
- Powieść historyczna
- Proza
- Przygodowa
- Publicystyka
- Reportaż
- Romans i literatura obyczajowa
- Sensacja
- Thriller, Horror
- Wywiady i wspomnienia
-
Nauki przyrodnicze
-
Nauki społeczne
-
Podręczniki szkolne
-
Popularnonaukowe i akademickie
- Archeologia
- Bibliotekoznawstwo
- Filmoznawstwo
- Filologia
- Filologia polska
- Filozofia
- Finanse i bankowość
- Geografia
- Gospodarka
- Handel. Gospodarka światowa
- Historia i archeologia
- Historia sztuki i architektury
- Kulturoznawstwo
- Lingwistyka
- Literaturoznawstwo
- Logistyka
- Matematyka
- Medycyna
- Nauki humanistyczne
- Pedagogika
- Pomoce naukowe
- Popularnonaukowa
- Pozostałe
- Psychologia
- Socjologia
- Teatrologia
- Teologia
- Teorie i nauki ekonomiczne
- Transport i spedycja
- Wychowanie fizyczne
- Zarządzanie i marketing
-
Poradniki
-
Poradniki do gier
-
Poradniki zawodowe i specjalistyczne
-
Prawo
- BHP
- Historia
- Kodeks drogowy. Prawo jazdy
- Nauki prawne
- Ochrona zdrowia
- Ogólne, kompendium wiedzy
- Podręczniki akademickie
- Pozostałe
- Prawo budowlane i lokalowe
- Prawo cywilne
- Prawo finansowe
- Prawo gospodarcze
- Prawo gospodarcze i handlowe
- Prawo karne
- Prawo karne. Przestępstwa karne. Kryminologia
- Prawo międzynarodowe
- Prawo międzynarodowe i zagraniczne
- Prawo ochrony zdrowia
- Prawo oświatowe
- Prawo podatkowe
- Prawo pracy i ubezpieczeń społecznych
- Prawo publiczne, konstytucyjne i administracyjne
- Prawo rodzinne i opiekuńcze
- Prawo rolne
- Prawo socjalne, prawo pracy
- Prawo Unii Europejskiej
- Przemysł
- Rolne i ochrona środowiska
- Słowniki i encyklopedie
- Zamówienia publiczne
- Zarządzanie
-
Przewodniki i podróże
- Afryka
- Albumy
- Ameryka Południowa
- Ameryka Środkowa i Północna
- Australia, Nowa Zelandia, Oceania
- Austria
- Azja
- Bałkany
- Bliski Wschód
- Bułgaria
- Chiny
- Chorwacja
- Czechy
- Dania
- Egipt
- Estonia
- Europa
- Francja
- Góry
- Grecja
- Hiszpania
- Holandia
- Islandia
- Litwa
- Łotwa
- Mapy, Plany miast, Atlasy
- Miniprzewodniki
- Niemcy
- Norwegia
- Podróże aktywne
- Polska
- Portugalia
- Pozostałe
- Przewodniki po hotelach i restauracjach
- Rosja
- Rumunia
- Słowacja
- Słowenia
- Szwajcaria
- Szwecja
- Świat
- Turcja
- Ukraina
- Węgry
- Wielka Brytania
- Włochy
-
Psychologia
- Filozofie życiowe
- Kompetencje psychospołeczne
- Komunikacja międzyludzka
- Mindfulness
- Ogólne
- Perswazja i NLP
- Psychologia akademicka
- Psychologia duszy i umysłu
- Psychologia pracy
- Relacje i związki
- Rodzicielstwo i psychologia dziecka
- Rozwiązywanie problemów
- Rozwój intelektualny
- Sekret
- Seksualność
- Uwodzenie
- Wygląd i wizerunek
- Życiowe filozofie
-
Religia
-
Sport, fitness, diety
-
Technika i mechanika
Audiobooki
-
Biznes i ekonomia
- Bitcoin
- Bizneswoman
- Coaching
- Controlling
- E-biznes
- Ekonomia
- Finanse
- Giełda i inwestycje
- Kompetencje osobiste
- Komunikacja i negocjacje
- Mała firma
- Marketing
- Motywacja
- Nieruchomości
- Perswazja i NLP
- Podatki
- Poradniki
- Prezentacje
- Przywództwo
- Public Relation
- Sekret
- Social Media
- Sprzedaż
- Start-up
- Twoja kariera
- Zarządzanie
- Zarządzanie projektami
- Zasoby ludzkie (HR)
-
Dla dzieci
-
Dla młodzieży
-
Edukacja
-
Encyklopedie, słowniki
-
Historia
-
Informatyka
-
Inne
-
Języki obce
-
Kultura i sztuka
-
Lektury szkolne
-
Literatura
- Antologie
- Ballada
- Biografie i autobiografie
- Dla dorosłych
- Dramat
- Dzienniki, pamiętniki, listy
- Epos, epopeja
- Esej
- Fantastyka i science-fiction
- Felietony
- Fikcja
- Humor, satyra
- Inne
- Klasyczna
- Kryminał
- Literatura faktu
- Literatura piękna
- Mity i legendy
- Nobliści
- Nowele
- Obyczajowa
- Okultyzm i magia
- Opowiadania
- Pamiętniki
- Podróże
- Poezja
- Polityka
- Popularnonaukowa
- Powieść
- Powieść historyczna
- Proza
- Przygodowa
- Publicystyka
- Reportaż
- Romans i literatura obyczajowa
- Sensacja
- Thriller, Horror
- Wywiady i wspomnienia
-
Nauki przyrodnicze
-
Nauki społeczne
-
Popularnonaukowe i akademickie
-
Poradniki
-
Poradniki zawodowe i specjalistyczne
-
Prawo
-
Przewodniki i podróże
-
Psychologia
- Filozofie życiowe
- Komunikacja międzyludzka
- Mindfulness
- Ogólne
- Perswazja i NLP
- Psychologia akademicka
- Psychologia duszy i umysłu
- Psychologia pracy
- Relacje i związki
- Rodzicielstwo i psychologia dziecka
- Rozwiązywanie problemów
- Rozwój intelektualny
- Sekret
- Seksualność
- Uwodzenie
- Wygląd i wizerunek
- Życiowe filozofie
-
Religia
-
Sport, fitness, diety
-
Technika i mechanika
Kursy video
-
Bazy danych
-
Big Data
-
Biznes, ekonomia i marketing
-
Cyberbezpieczeństwo
-
Data Science
-
DevOps
-
Dla dzieci
-
Elektronika
-
Grafika/Wideo/CAX
-
Gry
-
Microsoft Office
-
Narzędzia programistyczne
-
Programowanie
-
Rozwój osobisty
-
Sieci komputerowe
-
Systemy operacyjne
-
Testowanie oprogramowania
-
Urządzenia mobilne
-
UX/UI
-
Web development
-
Zarządzanie
Podcasty
- Ebooki
- Programowanie
- Python
- Hands-On Data Preprocessing in Python. Learn how to effectively prepare data for successful data analytics
Szczegóły ebooka
Zaloguj się, jeśli jesteś zainteresowany treścią pozycji.
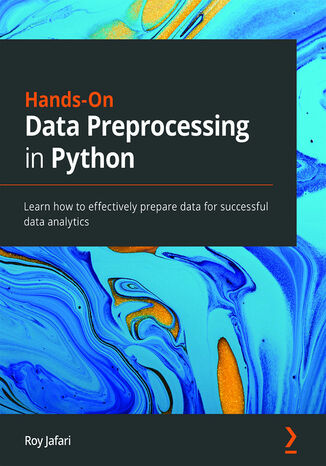
Hands-On Data Preprocessing in Python. Learn how to effectively prepare data for successful data analytics
Ebook
Hands-On Data Preprocessing is a primer on the best data cleaning and preprocessing techniques, written by an expert who’s developed college-level courses on data preprocessing and related subjects.
With this book, you’ll be equipped with the optimum data preprocessing techniques from multiple perspectives, ensuring that you get the best possible insights from your data.
You'll learn about different technical and analytical aspects of data preprocessing – data collection, data cleaning, data integration, data reduction, and data transformation – and get to grips with implementing them using the open source Python programming environment.
The hands-on examples and easy-to-follow chapters will help you gain a comprehensive articulation of data preprocessing, its whys and hows, and identify opportunities where data analytics could lead to more effective decision making. As you progress through the chapters, you’ll also understand the role of data management systems and technologies for effective analytics and how to use APIs to pull data.
By the end of this Python data preprocessing book, you'll be able to use Python to read, manipulate, and analyze data; perform data cleaning, integration, reduction, and transformation techniques, and handle outliers or missing values to effectively prepare data for analytic tools.
With this book, you’ll be equipped with the optimum data preprocessing techniques from multiple perspectives, ensuring that you get the best possible insights from your data.
You'll learn about different technical and analytical aspects of data preprocessing – data collection, data cleaning, data integration, data reduction, and data transformation – and get to grips with implementing them using the open source Python programming environment.
The hands-on examples and easy-to-follow chapters will help you gain a comprehensive articulation of data preprocessing, its whys and hows, and identify opportunities where data analytics could lead to more effective decision making. As you progress through the chapters, you’ll also understand the role of data management systems and technologies for effective analytics and how to use APIs to pull data.
By the end of this Python data preprocessing book, you'll be able to use Python to read, manipulate, and analyze data; perform data cleaning, integration, reduction, and transformation techniques, and handle outliers or missing values to effectively prepare data for analytic tools.
- 1. Review of the Core Modules of NumPy and Pandas
- 2. Review of Another Core Module - Matplotlib
- 3. Data – What Is It Really?
- 4. Databases
- 5. Data Visualization
- 6. Prediction
- 7. Classification
- 8. Clustering Analysis
- 9. Data Cleaning Level I - Cleaning Up the Table
- 10. Data Cleaning Level II - Unpacking, Restructuring, and Reformulating the Table
- 11. Data Cleaning Level III- Missing Values, Outliers, and Errors
- 12. Data Fusion and Data Integration
- 13. Data Reduction
- 14. Data Transformation and Massaging
- 15. Case Study 1 - Mental Health in Tech
- 16. Case Study 2 - Predicting COVID-19 Hospitalizations
- 17. Case Study 3: United States Counties Clustering Analysis
- 18. Summary, Practice Case Studies, and Conclusions
- Tytuł: Hands-On Data Preprocessing in Python. Learn how to effectively prepare data for successful data analytics
- Autor: Roy Jafari
- Tytuł oryginału: Hands-On Data Preprocessing in Python. Learn how to effectively prepare data for successful data analytics
- ISBN: 9781801079952, 9781801079952
- Data wydania: 2022-01-21
- Format: Ebook
- Identyfikator pozycji: e_2t1j
- Wydawca: Packt Publishing