Kategorie
Ebooki
-
Biznes i ekonomia
- Bitcoin
- Bizneswoman
- Coaching
- Controlling
- E-biznes
- Ekonomia
- Finanse
- Giełda i inwestycje
- Kompetencje osobiste
- Komputer w biurze
- Komunikacja i negocjacje
- Mała firma
- Marketing
- Motywacja
- Multimedialne szkolenia
- Nieruchomości
- Perswazja i NLP
- Podatki
- Polityka społeczna
- Poradniki
- Prezentacje
- Przywództwo
- Public Relation
- Raporty, analizy
- Sekret
- Social Media
- Sprzedaż
- Start-up
- Twoja kariera
- Zarządzanie
- Zarządzanie projektami
- Zasoby ludzkie (HR)
-
Dla dzieci
-
Dla młodzieży
-
Edukacja
-
Encyklopedie, słowniki
-
E-prasa
- Architektura i wnętrza
- BHP
- Biznes i Ekonomia
- Dom i ogród
- E-Biznes
- Ekonomia i finanse
- Finanse
- Finanse osobiste
- Firma
- Fotografia
- Informatyka
- Kadry i płace
- Kobieca
- Komputery, Excel
- Księgowość
- Kultura i literatura
- Naukowe i akademickie
- Ochrona środowiska
- Opiniotwórcze
- Oświata
- Podatki
- Podróże
- Psychologia
- Religia
- Rolnictwo
- Rynek książki i prasy
- Transport i Spedycja
- Zdrowie i uroda
-
Historia
-
Informatyka
- Aplikacje biurowe
- Bazy danych
- Bioinformatyka
- Biznes IT
- CAD/CAM
- Digital Lifestyle
- DTP
- Elektronika
- Fotografia cyfrowa
- Grafika komputerowa
- Gry
- Hacking
- Hardware
- IT w ekonomii
- Pakiety naukowe
- Podręczniki szkolne
- Podstawy komputera
- Programowanie
- Programowanie mobilne
- Serwery internetowe
- Sieci komputerowe
- Start-up
- Systemy operacyjne
- Sztuczna inteligencja
- Technologia dla dzieci
- Webmasterstwo
-
Inne
-
Języki obce
-
Kultura i sztuka
-
Lektury szkolne
-
Literatura
- Antologie
- Ballada
- Biografie i autobiografie
- Dla dorosłych
- Dramat
- Dzienniki, pamiętniki, listy
- Epos, epopeja
- Esej
- Fantastyka i science-fiction
- Felietony
- Fikcja
- Humor, satyra
- Inne
- Klasyczna
- Kryminał
- Literatura faktu
- Literatura piękna
- Mity i legendy
- Nobliści
- Nowele
- Obyczajowa
- Okultyzm i magia
- Opowiadania
- Pamiętniki
- Podróże
- Poemat
- Poezja
- Polityka
- Popularnonaukowa
- Powieść
- Powieść historyczna
- Proza
- Przygodowa
- Publicystyka
- Reportaż
- Romans i literatura obyczajowa
- Sensacja
- Thriller, Horror
- Wywiady i wspomnienia
-
Nauki przyrodnicze
-
Nauki społeczne
-
Podręczniki szkolne
-
Popularnonaukowe i akademickie
- Archeologia
- Bibliotekoznawstwo
- Filmoznawstwo
- Filologia
- Filologia polska
- Filozofia
- Finanse i bankowość
- Geografia
- Gospodarka
- Handel. Gospodarka światowa
- Historia i archeologia
- Historia sztuki i architektury
- Kulturoznawstwo
- Lingwistyka
- Literaturoznawstwo
- Logistyka
- Matematyka
- Medycyna
- Nauki humanistyczne
- Pedagogika
- Pomoce naukowe
- Popularnonaukowa
- Pozostałe
- Psychologia
- Socjologia
- Teatrologia
- Teologia
- Teorie i nauki ekonomiczne
- Transport i spedycja
- Wychowanie fizyczne
- Zarządzanie i marketing
-
Poradniki
-
Poradniki do gier
-
Poradniki zawodowe i specjalistyczne
-
Prawo
- BHP
- Historia
- Kodeks drogowy. Prawo jazdy
- Nauki prawne
- Ochrona zdrowia
- Ogólne, kompendium wiedzy
- Podręczniki akademickie
- Pozostałe
- Prawo budowlane i lokalowe
- Prawo cywilne
- Prawo finansowe
- Prawo gospodarcze
- Prawo gospodarcze i handlowe
- Prawo karne
- Prawo karne. Przestępstwa karne. Kryminologia
- Prawo międzynarodowe
- Prawo międzynarodowe i zagraniczne
- Prawo ochrony zdrowia
- Prawo oświatowe
- Prawo podatkowe
- Prawo pracy i ubezpieczeń społecznych
- Prawo publiczne, konstytucyjne i administracyjne
- Prawo rodzinne i opiekuńcze
- Prawo rolne
- Prawo socjalne, prawo pracy
- Prawo Unii Europejskiej
- Przemysł
- Rolne i ochrona środowiska
- Słowniki i encyklopedie
- Zamówienia publiczne
- Zarządzanie
-
Przewodniki i podróże
- Afryka
- Albumy
- Ameryka Południowa
- Ameryka Środkowa i Północna
- Australia, Nowa Zelandia, Oceania
- Austria
- Azja
- Bałkany
- Bliski Wschód
- Bułgaria
- Chiny
- Chorwacja
- Czechy
- Dania
- Egipt
- Estonia
- Europa
- Francja
- Góry
- Grecja
- Hiszpania
- Holandia
- Islandia
- Litwa
- Łotwa
- Mapy, Plany miast, Atlasy
- Miniprzewodniki
- Niemcy
- Norwegia
- Podróże aktywne
- Polska
- Portugalia
- Pozostałe
- Przewodniki po hotelach i restauracjach
- Rosja
- Rumunia
- Słowacja
- Słowenia
- Szwajcaria
- Szwecja
- Świat
- Turcja
- Ukraina
- Węgry
- Wielka Brytania
- Włochy
-
Psychologia
- Filozofie życiowe
- Kompetencje psychospołeczne
- Komunikacja międzyludzka
- Mindfulness
- Ogólne
- Perswazja i NLP
- Psychologia akademicka
- Psychologia duszy i umysłu
- Psychologia pracy
- Relacje i związki
- Rodzicielstwo i psychologia dziecka
- Rozwiązywanie problemów
- Rozwój intelektualny
- Sekret
- Seksualność
- Uwodzenie
- Wygląd i wizerunek
- Życiowe filozofie
-
Religia
-
Sport, fitness, diety
-
Technika i mechanika
Audiobooki
-
Biznes i ekonomia
- Bitcoin
- Bizneswoman
- Coaching
- Controlling
- E-biznes
- Ekonomia
- Finanse
- Giełda i inwestycje
- Kompetencje osobiste
- Komunikacja i negocjacje
- Mała firma
- Marketing
- Motywacja
- Nieruchomości
- Perswazja i NLP
- Podatki
- Polityka społeczna
- Poradniki
- Prezentacje
- Przywództwo
- Public Relation
- Sekret
- Social Media
- Sprzedaż
- Start-up
- Twoja kariera
- Zarządzanie
- Zarządzanie projektami
- Zasoby ludzkie (HR)
-
Dla dzieci
-
Dla młodzieży
-
Edukacja
-
Encyklopedie, słowniki
-
E-prasa
-
Historia
-
Informatyka
-
Inne
-
Języki obce
-
Kultura i sztuka
-
Lektury szkolne
-
Literatura
- Antologie
- Ballada
- Biografie i autobiografie
- Dla dorosłych
- Dramat
- Dzienniki, pamiętniki, listy
- Epos, epopeja
- Esej
- Fantastyka i science-fiction
- Felietony
- Fikcja
- Humor, satyra
- Inne
- Klasyczna
- Kryminał
- Literatura faktu
- Literatura piękna
- Mity i legendy
- Nobliści
- Nowele
- Obyczajowa
- Okultyzm i magia
- Opowiadania
- Pamiętniki
- Podróże
- Poezja
- Polityka
- Popularnonaukowa
- Powieść
- Powieść historyczna
- Proza
- Przygodowa
- Publicystyka
- Reportaż
- Romans i literatura obyczajowa
- Sensacja
- Thriller, Horror
- Wywiady i wspomnienia
-
Nauki przyrodnicze
-
Nauki społeczne
-
Popularnonaukowe i akademickie
-
Poradniki
-
Poradniki zawodowe i specjalistyczne
-
Prawo
-
Przewodniki i podróże
-
Psychologia
- Filozofie życiowe
- Komunikacja międzyludzka
- Mindfulness
- Ogólne
- Perswazja i NLP
- Psychologia akademicka
- Psychologia duszy i umysłu
- Psychologia pracy
- Relacje i związki
- Rodzicielstwo i psychologia dziecka
- Rozwiązywanie problemów
- Rozwój intelektualny
- Sekret
- Seksualność
- Uwodzenie
- Wygląd i wizerunek
- Życiowe filozofie
-
Religia
-
Sport, fitness, diety
-
Technika i mechanika
Kursy video
-
Bazy danych
-
Big Data
-
Biznes, ekonomia i marketing
-
Cyberbezpieczeństwo
-
Data Science
-
DevOps
-
Dla dzieci
-
Elektronika
-
Grafika/Wideo/CAX
-
Gry
-
Microsoft Office
-
Narzędzia programistyczne
-
Programowanie
-
Rozwój osobisty
-
Sieci komputerowe
-
Systemy operacyjne
-
Testowanie oprogramowania
-
Urządzenia mobilne
-
UX/UI
-
Web development
-
Zarządzanie
Podcasty
- Ebooki
- Popularnonaukowe i akademickie
- Matematyka
- Machine Learning with R. R gives you access to the cutting-edge software you need to prepare data for machine learning. No previous knowledge required – this book will take you methodically through every stage of applying machine learning
Szczegóły ebooka
Zaloguj się, jeśli jesteś zainteresowany treścią pozycji.
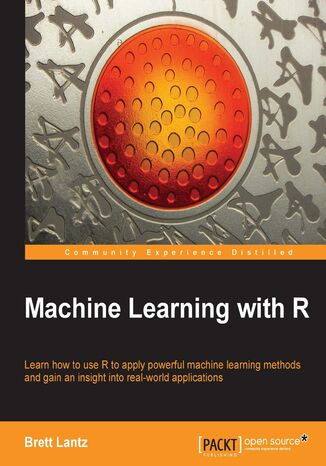
Machine Learning with R. R gives you access to the cutting-edge software you need to prepare data for machine learning. No previous knowledge required – this book will take you methodically through every stage of applying machine learning
Ebook
Machine learning, at its core, is concerned with transforming data into actionable knowledge. This fact makes machine learning well-suited to the present-day era of big data and data science. Given the growing prominence of R—a cross-platform, zero-cost statistical programming environment—there has never been a better time to start applying machine learning. Whether you are new to data science or a veteran, machine learning with R offers a powerful set of methods for quickly and easily gaining insight from your data.
Machine Learning with R is a practical tutorial that uses hands-on examples to step through real-world application of machine learning. Without shying away from the technical details, we will explore Machine Learning with R using clear and practical examples. Well-suited to machine learning beginners or those with experience. Explore R to find the answer to all of your questions.
How can we use machine learning to transform data into action? Using practical examples, we will explore how to prepare data for analysis, choose a machine learning method, and measure the success of the process.
We will learn how to apply machine learning methods to a variety of common tasks including classification, prediction, forecasting, market basket analysis, and clustering. By applying the most effective machine learning methods to real-world problems, you will gain hands-on experience that will transform the way you think about data.
Machine Learning with R will provide you with the analytical tools you need to quickly gain insight from complex data.
Machine Learning with R is a practical tutorial that uses hands-on examples to step through real-world application of machine learning. Without shying away from the technical details, we will explore Machine Learning with R using clear and practical examples. Well-suited to machine learning beginners or those with experience. Explore R to find the answer to all of your questions.
How can we use machine learning to transform data into action? Using practical examples, we will explore how to prepare data for analysis, choose a machine learning method, and measure the success of the process.
We will learn how to apply machine learning methods to a variety of common tasks including classification, prediction, forecasting, market basket analysis, and clustering. By applying the most effective machine learning methods to real-world problems, you will gain hands-on experience that will transform the way you think about data.
Machine Learning with R will provide you with the analytical tools you need to quickly gain insight from complex data.
- Machine Learning with R
- Table of Contents
- Machine Learning with R
- Credits
- About the Author
- About the Reviewers
- www.PacktPub.com
- Support files, eBooks, discount offers and more
- Why Subscribe?
- Free Access for Packt account holders
- Support files, eBooks, discount offers and more
- Preface
- What this book covers
- What you need for this book
- Who this book is for
- Conventions
- Reader feedback
- Customer support
- Downloading the example code
- Errata
- Piracy
- Questions
- 1. Introducing Machine Learning
- The origins of machine learning
- Uses and abuses of machine learning
- Ethical considerations
- How do machines learn?
- Abstraction and knowledge representation
- Generalization
- Assessing the success of learning
- Steps to apply machine learning to your data
- Choosing a machine learning algorithm
- Thinking about the input data
- Thinking about types of machine learning algorithms
- Matching your data to an appropriate algorithm
- Using R for machine learning
- Installing and loading R packages
- Installing an R package
- Installing a package using the point-and-click interface
- Loading an R package
- Installing and loading R packages
- Summary
- 2. Managing and Understanding Data
- R data structures
- Vectors
- Factors
- Lists
- Data frames
- Matrixes and arrays
- Managing data with R
- Saving and loading R data structures
- Importing and saving data from CSV files
- Importing data from SQL databases
- Exploring and understanding data
- Exploring the structure of data
- Exploring numeric variables
- Measuring the central tendency mean and median
- Measuring spread quartiles and the five-number summary
- Visualizing numeric variables boxplots
- Visualizing numeric variables histograms
- Understanding numeric data uniform and normal distributions
- Measuring spread variance and standard deviation
- Exploring categorical variables
- Measuring the central tendency the mode
- Exploring relationships between variables
- Visualizing relationships scatterplots
- Examining relationships two-way cross-tabulations
- Summary
- 3. Lazy Learning Classification Using Nearest Neighbors
- Understanding classification using nearest neighbors
- The kNN algorithm
- Calculating distance
- Choosing an appropriate k
- Preparing data for use with kNN
- Why is the kNN algorithm lazy?
- The kNN algorithm
- Diagnosing breast cancer with the kNN algorithm
- Step 1 collecting data
- Step 2 exploring and preparing the data
- Transformation normalizing numeric data
- Data preparation creating training and test datasets
- Step 3 training a model on the data
- Step 4 evaluating model performance
- Step 5 improving model performance
- Transformation z-score standardization
- Testing alternative values of k
- Summary
- Understanding classification using nearest neighbors
- 4. Probabilistic Learning Classification Using Naive Bayes
- Understanding naive Bayes
- Basic concepts of Bayesian methods
- Probability
- Joint probability
- Conditional probability with Bayes theorem
- The naive Bayes algorithm
- The naive Bayes classification
- The Laplace estimator
- Using numeric features with naive Bayes
- Basic concepts of Bayesian methods
- Example filtering mobile phone spam with the naive Bayes algorithm
- Step 1 collecting data
- Step 2 exploring and preparing the data
- Data preparation processing text data for analysis
- Data preparation creating training and test datasets
- Visualizing text data word clouds
- Data preparation creating indicator features for frequent words
- Step 3 training a model on the data
- Step 4 evaluating model performance
- Step 5 improving model performance
- Summary
- Understanding naive Bayes
- 5. Divide and Conquer Classification Using Decision Trees and Rules
- Understanding decision trees
- Divide and conquer
- The C5.0 decision tree algorithm
- Choosing the best split
- Pruning the decision tree
- Example identifying risky bank loans using C5.0 decision trees
- Step 1 collecting data
- Step 2 exploring and preparing the data
- Data preparation creating random training and test datasets
- Step 3 training a model on the data
- Step 4 evaluating model performance
- Step 5 improving model performance
- Boosting the accuracy of decision trees
- Making some mistakes more costly than others
- Understanding classification rules
- Separate and conquer
- The One Rule algorithm
- The RIPPER algorithm
- Rules from decision trees
- Example identifying poisonous mushrooms with rule learners
- Step 1 collecting data
- Step 2 exploring and preparing the data
- Step 3 training a model on the data
- Step 4 evaluating model performance
- Step 5 improving model performance
- Summary
- Understanding decision trees
- 6. Forecasting Numeric Data Regression Methods
- Understanding regression
- Simple linear regression
- Ordinary least squares estimation
- Correlations
- Multiple linear regression
- Example predicting medical expenses using linear regression
- Step 1 collecting data
- Step 2 exploring and preparing the data
- Exploring relationships among features the correlation matrix
- Visualizing relationships among features the scatterplot matrix
- Step 3 training a model on the data
- Step 4 evaluating model performance
- Step 5 improving model performance
- Model specification adding non-linear relationships
- Transformation converting a numeric variable to a binary indicator
- Model specification adding interaction effects
- Putting it all together an improved regression model
- Understanding regression trees and model trees
- Adding regression to trees
- Example estimating the quality of wines with regression trees and model trees
- Step 1 collecting data
- Step 2 exploring and preparing the data
- Step 3 training a model on the data
- Visualizing decision trees
- Step 4 evaluating model performance
- Measuring performance with mean absolute error
- Step 5 improving model performance
- Summary
- Understanding regression
- 7. Black Box Methods Neural Networks and Support Vector Machines
- Understanding neural networks
- From biological to artificial neurons
- Activation functions
- Network topology
- The number of layers
- The direction of information travel
- The number of nodes in each layer
- Training neural networks with backpropagation
- Modeling the strength of concrete with ANNs
- Step 1 collecting data
- Step 2 exploring and preparing the data
- Step 3 training a model on the data
- Step 4 evaluating model performance
- Step 5 improving model performance
- Understanding Support Vector Machines
- Classification with hyperplanes
- Finding the maximum margin
- The case of linearly separable data
- The case of non-linearly separable data
- Using kernels for non-linear spaces
- Performing OCR with SVMs
- Step 1 collecting data
- Step 2 exploring and preparing the data
- Step 3 training a model on the data
- Step 4 evaluating model performance
- Step 5 improving model performance
- Summary
- Understanding neural networks
- 8. Finding Patterns Market Basket Analysis Using Association Rules
- Understanding association rules
- The Apriori algorithm for association rule learning
- Measuring rule interest support and confidence
- Building a set of rules with the Apriori principle
- The Apriori algorithm for association rule learning
- Example identifying frequently purchased groceries with association rules
- Step 1 collecting data
- Step 2 exploring and preparing the data
- Data preparation creating a sparse matrix for transaction data
- Visualizing item support item frequency plots
- Visualizing transaction data plotting the sparse matrix
- Step 3 training a model on the data
- Step 4 evaluating model performance
- Step 5 improving model performance
- Sorting the set of association rules
- Taking subsets of association rules
- Saving association rules to a file or data frame
- Summary
- Understanding association rules
- 9. Finding Groups of Data Clustering with k-means
- Understanding clustering
- Clustering as a machine learning task
- The k-means algorithm for clustering
- Using distance to assign and update clusters
- Choosing the appropriate number of clusters
- Finding teen market segments using k-means clustering
- Step 1 collecting data
- Step 2 exploring and preparing the data
- Data preparation dummy coding missing values
- Data preparation imputing missing values
- Step 3 training a model on the data
- Step 4 evaluating model performance
- Step 5 improving model performance
- Summary
- Understanding clustering
- 10. Evaluating Model Performance
- Measuring performance for classification
- Working with classification prediction data in R
- A closer look at confusion matrices
- Using confusion matrices to measure performance
- Beyond accuracy other measures of performance
- The kappa statistic
- Sensitivity and specificity
- Precision and recall
- The F-measure
- Visualizing performance tradeoffs
- ROC curves
- Estimating future performance
- The holdout method
- Cross-validation
- Bootstrap sampling
- Summary
- Measuring performance for classification
- 11. Improving Model Performance
- Tuning stock models for better performance
- Using caret for automated parameter tuning
- Creating a simple tuned model
- Customizing the tuning process
- Using caret for automated parameter tuning
- Improving model performance with meta-learning
- Understanding ensembles
- Bagging
- Boosting
- Random forests
- Training random forests
- Evaluating random forest performance
- Summary
- Tuning stock models for better performance
- 12. Specialized Machine Learning Topics
- Working with specialized data
- Getting data from the Web with the RCurl package
- Reading and writing XML with the XML package
- Reading and writing JSON with the rjson package
- Reading and writing Microsoft Excel spreadsheets using xlsx
- Working with bioinformatics data
- Working with social network data and graph data
- Improving the performance of R
- Managing very large datasets
- Making data frames faster with data.table
- Creating disk-based data frames with ff
- Using massive matrices with bigmemory
- Learning faster with parallel computing
- Measuring execution time
- Working in parallel with foreach
- Using a multitasking operating system with multicore
- Networking multiple workstations with snow and snowfall
- Parallel cloud computing with MapReduce and Hadoop
- GPU computing
- Deploying optimized learning algorithms
- Building bigger regression models with biglm
- Growing bigger and faster random forests with bigrf
- Training and evaluating models in parallel with caret
- Managing very large datasets
- Summary
- Working with specialized data
- Index
- Tytuł: Machine Learning with R. R gives you access to the cutting-edge software you need to prepare data for machine learning. No previous knowledge required ‚Äì this book will take you methodically through every stage of applying machine learning
- Autor: Brett Lantz
- Tytuł oryginału: Machine Learning with R. R gives you access to the cutting-edge software you need to prepare data for machine learning. No previous knowledge required ‚Äì this book will take you methodically through every stage of applying machine learning.
- ISBN: 9781782162155, 9781782162155
- Data wydania: 2013-10-25
- Format: Ebook
- Identyfikator pozycji: e_3bnp
- Wydawca: Packt Publishing