Categories
Ebooks
-
Business and economy
- Bitcoin
- Businesswoman
- Coaching
- Controlling
- E-business
- Economy
- Finances
- Stocks and investments
- Personal competence
- Computer in the office
- Communication and negotiation
- Small company
- Marketing
- Motivation
- Multimedia trainings
- Real estate
- Persuasion and NLP
- Taxes
- Social policy
- Guides
- Presentations
- Leadership
- Public Relation
- Reports, analyses
- Secret
- Social Media
- Sales
- Start-up
- Your career
- Management
- Project management
- Human Resources
-
For children
-
For youth
-
Education
-
Encyclopedias, dictionaries
-
E-press
- Architektura i wnętrza
- Health and Safety
- Biznes i Ekonomia
- Home and garden
- E-business
- Ekonomia i finanse
- Finances
- Personal finance
- Business
- Photography
- Computer science
- HR & Payroll
- For women
- Computers, Excel
- Accounts
- Culture and literature
- Scientific and academic
- Environmental protection
- Opinion-forming
- Education
- Taxes
- Travelling
- Psychology
- Religion
- Agriculture
- Book and press market
- Transport and Spedition
- Healthand beauty
-
History
-
Computer science
- Office applications
- Data bases
- Bioinformatics
- IT business
- CAD/CAM
- Digital Lifestyle
- DTP
- Electronics
- Digital photography
- Computer graphics
- Games
- Hacking
- Hardware
- IT w ekonomii
- Scientific software package
- School textbooks
- Computer basics
- Programming
- Mobile programming
- Internet servers
- Computer networks
- Start-up
- Operational systems
- Artificial intelligence
- Technology for children
- Webmastering
-
Other
-
Foreign languages
-
Culture and art
-
School reading books
-
Literature
- Antology
- Ballade
- Biographies and autobiographies
- For adults
- Dramas
- Diaries, memoirs, letters
- Epic, epopee
- Essay
- Fantasy and science fiction
- Feuilletons
- Work of fiction
- Humour and satire
- Other
- Classical
- Crime fiction
- Non-fiction
- Fiction
- Mity i legendy
- Nobelists
- Novellas
- Moral
- Okultyzm i magia
- Short stories
- Memoirs
- Travelling
- Narrative poetry
- Poetry
- Politics
- Popular science
- Novel
- Historical novel
- Prose
- Adventure
- Journalism, publicism
- Reportage novels
- Romans i literatura obyczajowa
- Sensational
- Thriller, Horror
- Interviews and memoirs
-
Natural sciences
-
Social sciences
-
School textbooks
-
Popular science and academic
- Archeology
- Bibliotekoznawstwo
- Cinema studies
- Philology
- Polish philology
- Philosophy
- Finanse i bankowość
- Geography
- Economy
- Trade. World economy
- History and archeology
- History of art and architecture
- Cultural studies
- Linguistics
- Literary studies
- Logistics
- Maths
- Medicine
- Humanities
- Pedagogy
- Educational aids
- Popular science
- Other
- Psychology
- Sociology
- Theatre studies
- Theology
- Economic theories and teachings
- Transport i spedycja
- Physical education
- Zarządzanie i marketing
-
Guides
-
Game guides
-
Professional and specialist guides
-
Law
- Health and Safety
- History
- Road Code. Driving license
- Law studies
- Healthcare
- General. Compendium of knowledge
- Academic textbooks
- Other
- Construction and local law
- Civil law
- Financial law
- Economic law
- Economic and trade law
- Criminal law
- Criminal law. Criminal offenses. Criminology
- International law
- International law
- Health care law
- Educational law
- Tax law
- Labor and social security law
- Public, constitutional and administrative law
- Family and Guardianship Code
- agricultural law
- Social law, labour law
- European Union law
- Industry
- Agricultural and environmental
- Dictionaries and encyclopedia
- Public procurement
- Management
-
Tourist guides and travel
- Africa
- Albums
- Southern America
- North and Central America
- Australia, New Zealand, Oceania
- Austria
- Asia
- Balkans
- Middle East
- Bulgary
- China
- Croatia
- The Czech Republic
- Denmark
- Egipt
- Estonia
- Europe
- France
- Mountains
- Greece
- Spain
- Holand
- Iceland
- Lithuania
- Latvia
- Mapy, Plany miast, Atlasy
- Mini travel guides
- Germany
- Norway
- Active travelling
- Poland
- Portugal
- Other
- Przewodniki po hotelach i restauracjach
- Russia
- Romania
- Slovakia
- Slovenia
- Switzerland
- Sweden
- World
- Turkey
- Ukraine
- Hungary
- Great Britain
- Italy
-
Psychology
- Philosophy of life
- Kompetencje psychospołeczne
- Interpersonal communication
- Mindfulness
- General
- Persuasion and NLP
- Academic psychology
- Psychology of soul and mind
- Work psychology
- Relacje i związki
- Parenting and children psychology
- Problem solving
- Intellectual growth
- Secret
- Sexapeal
- Seduction
- Appearance and image
- Philosophy of life
-
Religion
-
Sport, fitness, diets
-
Technology and mechanics
Audiobooks
-
Business and economy
- Bitcoin
- Businesswoman
- Coaching
- Controlling
- E-business
- Economy
- Finances
- Stocks and investments
- Personal competence
- Communication and negotiation
- Small company
- Marketing
- Motivation
- Real estate
- Persuasion and NLP
- Taxes
- Guides
- Presentations
- Leadership
- Public Relation
- Secret
- Social Media
- Sales
- Start-up
- Your career
- Management
- Project management
- Human Resources
-
For children
-
For youth
-
Education
-
Encyclopedias, dictionaries
-
E-press
-
History
-
Computer science
-
Other
-
Foreign languages
-
Culture and art
-
School reading books
-
Literature
- Antology
- Ballade
- Biographies and autobiographies
- For adults
- Dramas
- Diaries, memoirs, letters
- Epic, epopee
- Essay
- Fantasy and science fiction
- Feuilletons
- Work of fiction
- Humour and satire
- Other
- Classical
- Crime fiction
- Non-fiction
- Fiction
- Mity i legendy
- Nobelists
- Novellas
- Moral
- Okultyzm i magia
- Short stories
- Memoirs
- Travelling
- Poetry
- Politics
- Popular science
- Novel
- Historical novel
- Prose
- Adventure
- Journalism, publicism
- Reportage novels
- Romans i literatura obyczajowa
- Sensational
- Thriller, Horror
- Interviews and memoirs
-
Natural sciences
-
Social sciences
-
Popular science and academic
-
Guides
-
Professional and specialist guides
-
Law
-
Tourist guides and travel
-
Psychology
- Philosophy of life
- Interpersonal communication
- Mindfulness
- General
- Persuasion and NLP
- Academic psychology
- Psychology of soul and mind
- Work psychology
- Relacje i związki
- Parenting and children psychology
- Problem solving
- Intellectual growth
- Secret
- Sexapeal
- Seduction
- Appearance and image
- Philosophy of life
-
Religion
-
Sport, fitness, diets
-
Technology and mechanics
Videocourses
-
Data bases
-
Big Data
-
Biznes, ekonomia i marketing
-
Cybersecurity
-
Data Science
-
DevOps
-
For children
-
Electronics
-
Graphics/Video/CAX
-
Games
-
Microsoft Office
-
Development tools
-
Programming
-
Personal growth
-
Computer networks
-
Operational systems
-
Software testing
-
Mobile devices
-
UX/UI
-
Web development
-
Management
Podcasts
- Ebooks
- Big data
- Machine learning
- Machine Learning Security with Azure. Best practices for assessing, securing, and monitoring Azure Machine Learning workloads
E-book details
Log in, If you're interested in the contents of the item.
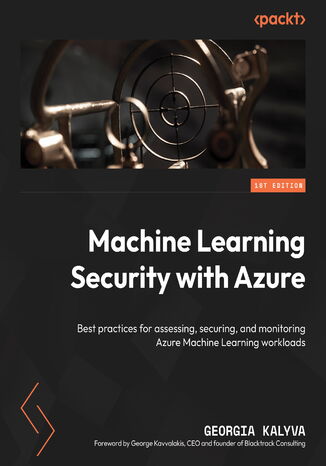
Machine Learning Security with Azure. Best practices for assessing, securing, and monitoring Azure Machine Learning workloads
Georgia Kalyva, George Kavvalakis
Ebook
With AI and machine learning (ML) models gaining popularity and integrating into more and more applications, it is more important than ever to ensure that models perform accurately and are not vulnerable to cyberattacks. However, attacks can target your data or environment as well. This book will help you identify security risks and apply the best practices to protect your assets on multiple levels, from data and models to applications and infrastructure.
This book begins by introducing what some common ML attacks are, how to identify your risks, and the industry standards and responsible AI principles you need to follow to gain an understanding of what you need to protect. Next, you will learn about the best practices to secure your assets. Starting with data protection and governance and then moving on to protect your infrastructure, you will gain insights into managing and securing your Azure ML workspace. This book introduces DevOps practices to automate your tasks securely and explains how to recover from ML attacks. Finally, you will learn how to set a security benchmark for your scenario and best practices to maintain and monitor your security posture.
By the end of this book, you’ll be able to implement best practices to assess and secure your ML assets throughout the Azure Machine Learning life cycle.
This book begins by introducing what some common ML attacks are, how to identify your risks, and the industry standards and responsible AI principles you need to follow to gain an understanding of what you need to protect. Next, you will learn about the best practices to secure your assets. Starting with data protection and governance and then moving on to protect your infrastructure, you will gain insights into managing and securing your Azure ML workspace. This book introduces DevOps practices to automate your tasks securely and explains how to recover from ML attacks. Finally, you will learn how to set a security benchmark for your scenario and best practices to maintain and monitor your security posture.
By the end of this book, you’ll be able to implement best practices to assess and secure your ML assets throughout the Azure Machine Learning life cycle.
- 1. Assessing the Vulnerability of Your Algorithms, Models, and AI Environments
- 2. Understanding the Most Common Machine Learning Attacks
- 3. Planning for Regulatory Compliance
- 4. Data Protection and Governance
- 5. Data Privacy and Responsible AI Best Practices
- 6. Managing and Securing Access
- 7. Managing and Securing Your Azure Machine Learning Workspace
- 8. Managing and Securing the MLOps Lifecycle
- 9. Logging, Monitoring, and Threat Detection
- 10. Setting a Security Baseline for Your Azure ML Workloads
- Title: Machine Learning Security with Azure. Best practices for assessing, securing, and monitoring Azure Machine Learning workloads
- Author: Georgia Kalyva, George Kavvalakis
- Original title: Machine Learning Security with Azure. Best practices for assessing, securing, and monitoring Azure Machine Learning workloads
- ISBN: 9781805123958, 9781805123958
- Date of issue: 2023-12-28
- Format: Ebook
- Item ID: e_3r11
- Publisher: Packt Publishing