Категорії
Електронні книги
-
Бізнес та економіка
- Біткойн
- Ділова жінка
- Коучинг
- Контроль
- Електронний бізнес
- Економіка
- Фінанси
- Фондова біржа та інвестиції
- Особисті компетенції
- Комп'ютер в офісі
- Комунікація та переговори
- Малий бізнес
- Маркетинг
- Мотивація
- Мультимедійне навчання
- Нерухомість
- Переконання та НЛП
- Податки
- Соціальна політика
- Порадники
- Презентації
- Лідерство
- Зв'язки з громадськістю
- Звіти, аналізи
- Секрет
- Соціальні засоби комунікації
- Продаж
- Стартап
- Ваша кар'єра
- Управління
- Управління проектами
- Людські ресурси (HR)
-
Для дітей
-
Для молоді
-
Освіта
-
Енциклопедії, словники
-
Електронна преса
- Architektura i wnętrza
- Безпека життєдіяльності
- Biznes i Ekonomia
- Будинок та сад
- Електронний бізнес
- Ekonomia i finanse
- Езотерика
- Фінанси
- Особисті фінанси
- Бізнес
- Фотографія
- Інформатика
- Відділ кадрів та оплата праці
- Для жінок
- Комп'ютери, Excel
- Бухгалтерія
- Культура та література
- Наукові та академічні
- Охорона навколишнього середовища
- Впливові
- Освіта
- Податки
- Подорожі
- Психологія
- Релігія
- Сільське господарство
- Ринок книг і преси
- Транспорт та спедиція
- Здоров'я та краса
-
Історія
-
Інформатика
- Офісні застосунки
- Бази даних
- Біоінформатика
- Бізнес ІТ
- CAD/CAM
- Digital Lifestyle
- DTP
- Електроніка
- Цифрова фотографія
- Комп'ютерна графіка
- Ігри
- Хакування
- Hardware
- IT w ekonomii
- Наукові пакети
- Шкільні підручники
- Основи комп'ютера
- Програмування
- Мобільне програмування
- Інтернет-сервери
- Комп'ютерні мережі
- Стартап
- Операційні системи
- Штучний інтелект
- Технологія для дітей
- Вебмайстерність
-
Інше
-
Іноземні мови
-
Культура та мистецтво
-
Шкільні читанки
-
Література
- Антології
- Балада
- Біографії та автобіографії
- Для дорослих
- Драми
- Журнали, щоденники, листи
- Епос, епопея
- Нарис
- Наукова фантастика та фантастика
- Фельєтони
- Художня література
- Гумор, сатира
- Інше
- Класичний
- Кримінальний роман
- Нехудожня література
- Художня література
- Mity i legendy
- Лауреати Нобелівської премії
- Новели
- Побутовий роман
- Okultyzm i magia
- Оповідання
- Спогади
- Подорожі
- Оповідна поезія
- Поезія
- Політика
- Науково-популярна
- Роман
- Історичний роман
- Проза
- Пригодницька
- Журналістика
- Роман-репортаж
- Romans i literatura obyczajowa
- Сенсація
- Трилер, жах
- Інтерв'ю та спогади
-
Природничі науки
-
Соціальні науки
-
Шкільні підручники
-
Науково-популярна та академічна
- Археологія
- Bibliotekoznawstwo
- Кінознавство / Теорія кіно
- Філологія
- Польська філологія
- Філософія
- Finanse i bankowość
- Географія
- Економіка
- Торгівля. Світова економіка
- Історія та археологія
- Історія мистецтва і архітектури
- Культурологія
- Мовознавство
- літературні студії
- Логістика
- Математика
- Ліки
- Гуманітарні науки
- Педагогіка
- Навчальні засоби
- Науково-популярна
- Інше
- Психологія
- Соціологія
- Театральні студії
- Богослов’я
- Економічні теорії та науки
- Transport i spedycja
- Фізичне виховання
- Zarządzanie i marketing
-
Порадники
-
Ігрові посібники
-
Професійні та спеціальні порадники
-
Юридична
- Безпека життєдіяльності
- Історія
- Дорожній кодекс. Водійські права
- Юридичні науки
- Охорона здоров'я
- Загальне, компендіум
- Академічні підручники
- Інше
- Закон про будівництво і житло
- Цивільне право
- Фінансове право
- Господарське право
- Господарське та комерційне право
- Кримінальний закон
- Кримінальне право. Кримінальні злочини. Кримінологія
- Міжнародне право
- Міжнародне та іноземне право
- Закон про охорону здоров'я
- Закон про освіту
- Податкове право
- Трудове право та законодавство про соціальне забезпечення
- Громадське, конституційне та адміністративне право
- Кодекс про шлюб і сім'ю
- Аграрне право
- Соціальне право, трудове право
- Законодавство Євросоюзу
- Промисловість
- Сільське господарство та захист навколишнього середовища
- Словники та енциклопедії
- Державні закупівлі
- Управління
-
Путівники та подорожі
- Африка
- Альбоми
- Південна Америка
- Центральна та Північна Америка
- Австралія, Нова Зеландія, Океанія
- Австрія
- Азії
- Балкани
- Близький Схід
- Болгарія
- Китай
- Хорватія
- Чеська Республіка
- Данія
- Єгипет
- Естонія
- Європа
- Франція
- Гори
- Греція
- Іспанія
- Нідерланди
- Ісландія
- Литва
- Латвія
- Mapy, Plany miast, Atlasy
- Мініпутівники
- Німеччина
- Норвегія
- Активні подорожі
- Польща
- Португалія
- Інше
- Przewodniki po hotelach i restauracjach
- Росія
- Румунія
- Словаччина
- Словенія
- Швейцарія
- Швеція
- Світ
- Туреччина
- Україна
- Угорщина
- Велика Британія
- Італія
-
Психологія
- Філософія життя
- Kompetencje psychospołeczne
- Міжособистісне спілкування
- Mindfulness
- Загальне
- Переконання та НЛП
- Академічна психологія
- Психологія душі та розуму
- Психологія праці
- Relacje i związki
- Батьківство та дитяча психологія
- Вирішення проблем
- Інтелектуальний розвиток
- Секрет
- Сексуальність
- Спокушання
- Зовнішній вигляд та імідж
- Філософія життя
-
Релігія
-
Спорт, фітнес, дієти
-
Техніка і механіка
Аудіокниги
-
Бізнес та економіка
- Біткойн
- Ділова жінка
- Коучинг
- Контроль
- Електронний бізнес
- Економіка
- Фінанси
- Фондова біржа та інвестиції
- Особисті компетенції
- Комунікація та переговори
- Малий бізнес
- Маркетинг
- Мотивація
- Нерухомість
- Переконання та НЛП
- Податки
- Соціальна політика
- Порадники
- Презентації
- Лідерство
- Зв'язки з громадськістю
- Секрет
- Соціальні засоби комунікації
- Продаж
- Стартап
- Ваша кар'єра
- Управління
- Управління проектами
- Людські ресурси (HR)
-
Для дітей
-
Для молоді
-
Освіта
-
Енциклопедії, словники
-
Електронна преса
-
Історія
-
Інформатика
-
Інше
-
Іноземні мови
-
Культура та мистецтво
-
Шкільні читанки
-
Література
- Антології
- Балада
- Біографії та автобіографії
- Для дорослих
- Драми
- Журнали, щоденники, листи
- Епос, епопея
- Нарис
- Наукова фантастика та фантастика
- Фельєтони
- Художня література
- Гумор, сатира
- Інше
- Класичний
- Кримінальний роман
- Нехудожня література
- Художня література
- Mity i legendy
- Лауреати Нобелівської премії
- Новели
- Побутовий роман
- Okultyzm i magia
- Оповідання
- Спогади
- Подорожі
- Поезія
- Політика
- Науково-популярна
- Роман
- Історичний роман
- Проза
- Пригодницька
- Журналістика
- Роман-репортаж
- Romans i literatura obyczajowa
- Сенсація
- Трилер, жах
- Інтерв'ю та спогади
-
Природничі науки
-
Соціальні науки
-
Науково-популярна та академічна
-
Порадники
-
Професійні та спеціальні порадники
-
Юридична
-
Путівники та подорожі
-
Психологія
- Філософія життя
- Міжособистісне спілкування
- Mindfulness
- Загальне
- Переконання та НЛП
- Академічна психологія
- Психологія душі та розуму
- Психологія праці
- Relacje i związki
- Батьківство та дитяча психологія
- Вирішення проблем
- Інтелектуальний розвиток
- Секрет
- Сексуальність
- Спокушання
- Зовнішній вигляд та імідж
- Філософія життя
-
Релігія
-
Спорт, фітнес, дієти
-
Техніка і механіка
Відеокурси
-
Бази даних
-
Big Data
-
Biznes, ekonomia i marketing
-
Кібербезпека
-
Data Science
-
DevOps
-
Для дітей
-
Електроніка
-
Графіка / Відео / CAX
-
Ігри
-
Microsoft Office
-
Інструменти розробки
-
Програмування
-
Особистісний розвиток
-
Комп'ютерні мережі
-
Операційні системи
-
Тестування програмного забезпечення
-
Мобільні пристрої
-
UX/UI
-
Веброзробка, Web development
-
Управління
Подкасти
- Електронні книги
- Big data (Великі дані)
- Машинне навчання
- Python: Deeper Insights into Machine Learning. Deeper Insights into Machine Learning
Деталі електронної книги
Увійти, Якщо вас цікавить зміст видання.
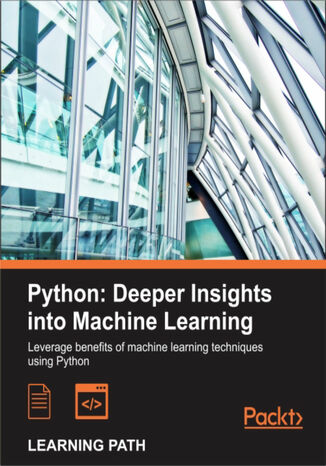
Python: Deeper Insights into Machine Learning. Deeper Insights into Machine Learning
David Julian, Sebastian Raschka, John Hearty
Eлектронна книга
Machine learning and predictive analytics are becoming one of the key strategies for unlocking growth in a challenging contemporary marketplace. It is one of the fastest growing trends in modern computing, and everyone wants to get into the field of machine learning. In order to obtain sufficient recognition in this field, one must be able to understand and design a machine learning system that serves the needs of a project.
The idea is to prepare a learning path that will help you to tackle the real-world complexities of modern machine learning with innovative and cutting-edge techniques. Also, it will give you a solid foundation in the machine learning design process, and enable you to build customized machine learning models to solve unique problems.
The course begins with getting your Python fundamentals nailed down. It focuses on answering the right questions that cove a wide range of powerful Python libraries, including scikit-learn Theano and Keras.After getting familiar with Python core concepts, it’s time to dive into the field of data science. You will further gain a solid foundation on the machine learning design and also learn to customize models for solving problems.
At a later stage, you will get a grip on more advanced techniques and acquire a broad set of powerful skills in the area of feature selection and feature engineering.
The idea is to prepare a learning path that will help you to tackle the real-world complexities of modern machine learning with innovative and cutting-edge techniques. Also, it will give you a solid foundation in the machine learning design process, and enable you to build customized machine learning models to solve unique problems.
The course begins with getting your Python fundamentals nailed down. It focuses on answering the right questions that cove a wide range of powerful Python libraries, including scikit-learn Theano and Keras.After getting familiar with Python core concepts, it’s time to dive into the field of data science. You will further gain a solid foundation on the machine learning design and also learn to customize models for solving problems.
At a later stage, you will get a grip on more advanced techniques and acquire a broad set of powerful skills in the area of feature selection and feature engineering.
- Python: Deeper Insights into Machine Learning
- Table of Contents
- Python: Deeper Insights into Machine Learning
- Python: Deeper Insights into Machine Learning
- Credits
- Preface
- What this learning path covers
- What you need for this learning path
- Who this learning path is for
- Reader feedback
- Customer support
- Downloading the example code
- Errata
- Piracy
- Questions
- 1. Module 1
- 1. Giving Computers the Ability to Learn from Data
- Building intelligent machines to transform data into knowledge
- The three different types of machine learning
- Making predictions about the future with supervised learning
- Classification for predicting class labels
- Regression for predicting continuous outcomes
- Solving interactive problems with reinforcement learning
- Discovering hidden structures with unsupervised learning
- Finding subgroups with clustering
- Dimensionality reduction for data compression
- Making predictions about the future with supervised learning
- An introduction to the basic terminology and notations
- A roadmap for building machine learning systems
- Preprocessing getting data into shape
- Training and selecting a predictive model
- Evaluating models and predicting unseen data instances
- Using Python for machine learning
- Installing Python packages
- Summary
- 2. Training Machine Learning Algorithms for Classification
- Artificial neurons a brief glimpse into the early history of machine learning
- Implementing a perceptron learning algorithm in Python
- Training a perceptron model on the Iris dataset
- Adaptive linear neurons and the convergence of learning
- Minimizing cost functions with gradient descent
- Implementing an Adaptive Linear Neuron in Python
- Large scale machine learning and stochastic gradient descent
- Summary
- 3. A Tour of Machine Learning Classifiers Using Scikit-learn
- Choosing a classification algorithm
- First steps with scikit-learn
- Training a perceptron via scikit-learn
- Modeling class probabilities via logistic regression
- Logistic regression intuition and conditional probabilities
- Learning the weights of the logistic cost function
- Training a logistic regression model with scikit-learn
- Tackling overfitting via regularization
- Maximum margin classification with support vector machines
- Maximum margin intuition
- Dealing with the nonlinearly separable case using slack variables
- Alternative implementations in scikit-learn
- Solving nonlinear problems using a kernel SVM
- Using the kernel trick to find separating hyperplanes in higher dimensional space
- Decision tree learning
- Maximizing information gain getting the most bang for the buck
- Building a decision tree
- Combining weak to strong learners via random forests
- K-nearest neighbors a lazy learning algorithm
- Summary
- 4. Building Good Training Sets Data Preprocessing
- Dealing with missing data
- Eliminating samples or features with missing values
- Imputing missing values
- Understanding the scikit-learn estimator API
- Handling categorical data
- Mapping ordinal features
- Encoding class labels
- Performing one-hot encoding on nominal features
- Partitioning a dataset in training and test sets
- Bringing features onto the same scale
- Selecting meaningful features
- Sparse solutions with L1 regularization
- Sequential feature selection algorithms
- Assessing feature importance with random forests
- Summary
- Dealing with missing data
- 5. Compressing Data via Dimensionality Reduction
- Unsupervised dimensionality reduction via principal component analysis
- Total and explained variance
- Feature transformation
- Principal component analysis in scikit-learn
- Supervised data compression via linear discriminant analysis
- Computing the scatter matrices
- Selecting linear discriminants for the new feature subspace
- Projecting samples onto the new feature space
- LDA via scikit-learn
- Using kernel principal component analysis for nonlinear mappings
- Kernel functions and the kernel trick
- Implementing a kernel principal component analysis in Python
- Example 1 separating half-moon shapes
- Example 2 separating concentric circles
- Projecting new data points
- Kernel principal component analysis in scikit-learn
- Summary
- Unsupervised dimensionality reduction via principal component analysis
- 6. Learning Best Practices for Model Evaluation and Hyperparameter Tuning
- Streamlining workflows with pipelines
- Loading the Breast Cancer Wisconsin dataset
- Combining transformers and estimators in a pipeline
- Using k-fold cross-validation to assess model performance
- The holdout method
- K-fold cross-validation
- Debugging algorithms with learning and validation curves
- Diagnosing bias and variance problems with learning curves
- Addressing overfitting and underfitting with validation curves
- Fine-tuning machine learning models via grid search
- Tuning hyperparameters via grid search
- Algorithm selection with nested cross-validation
- Looking at different performance evaluation metrics
- Reading a confusion matrix
- Optimizing the precision and recall of a classification model
- Plotting a receiver operating characteristic
- The scoring metrics for multiclass classification
- Summary
- Streamlining workflows with pipelines
- 7. Combining Different Models for Ensemble Learning
- Learning with ensembles
- Implementing a simple majority vote classifier
- Combining different algorithms for classification with majority vote
- Evaluating and tuning the ensemble classifier
- Bagging building an ensemble of classifiers from bootstrap samples
- Leveraging weak learners via adaptive boosting
- Summary
- 8. Applying Machine Learning to Sentiment Analysis
- Obtaining the IMDb movie review dataset
- Introducing the bag-of-words model
- Transforming words into feature vectors
- Assessing word relevancy via term frequency-inverse document frequency
- Cleaning text data
- Processing documents into tokens
- Training a logistic regression model for document classification
- Working with bigger data online algorithms and out-of-core learning
- Summary
- 9. Embedding a Machine Learning Model into a Web Application
- Serializing fitted scikit-learn estimators
- Setting up a SQLite database for data storage
- Developing a web application with Flask
- Our first Flask web application
- Form validation and rendering
- Turning the movie classifier into a web application
- Deploying the web application to a public server
- Updating the movie review classifier
- Summary
- 10. Predicting Continuous Target Variables with Regression Analysis
- Introducing a simple linear regression model
- Exploring the Housing Dataset
- Visualizing the important characteristics of a dataset
- Implementing an ordinary least squares linear regression model
- Solving regression for regression parameters with gradient descent
- Estimating the coefficient of a regression model via scikit-learn
- Fitting a robust regression model using RANSAC
- Evaluating the performance of linear regression models
- Using regularized methods for regression
- Turning a linear regression model into a curve polynomial regression
- Modeling nonlinear relationships in the Housing Dataset
- Dealing with nonlinear relationships using random forests
- Decision tree regression
- Random forest regression
- Summary
- 11. Working with Unlabeled Data Clustering Analysis
- Grouping objects by similarity using k-means
- K-means++
- Hard versus soft clustering
- Using the elbow method to find the optimal number of clusters
- Quantifying the quality of clustering via silhouette plots
- Organizing clusters as a hierarchical tree
- Performing hierarchical clustering on a distance matrix
- Attaching dendrograms to a heat map
- Applying agglomerative clustering via scikit-learn
- Locating regions of high density via DBSCAN
- Summary
- Grouping objects by similarity using k-means
- 12. Training Artificial Neural Networks for Image Recognition
- Modeling complex functions with artificial neural networks
- Single-layer neural network recap
- Introducing the multi-layer neural network architecture
- Activating a neural network via forward propagation
- Classifying handwritten digits
- Obtaining the MNIST dataset
- Implementing a multi-layer perceptron
- Training an artificial neural network
- Computing the logistic cost function
- Training neural networks via backpropagation
- Developing your intuition for backpropagation
- Debugging neural networks with gradient checking
- Convergence in neural networks
- Other neural network architectures
- Convolutional Neural Networks
- Recurrent Neural Networks
- A few last words about neural network implementation
- Summary
- Modeling complex functions with artificial neural networks
- 13. Parallelizing Neural Network Training with Theano
- Building, compiling, and running expressions with Theano
- What is Theano?
- First steps with Theano
- Configuring Theano
- Working with array structures
- Wrapping things up a linear regression example
- Choosing activation functions for feedforward neural networks
- Logistic function recap
- Estimating probabilities in multi-class classification via the softmax function
- Broadening the output spectrum by using a hyperbolic tangent
- Training neural networks efficiently using Keras
- Summary
- Building, compiling, and running expressions with Theano
- 1. Giving Computers the Ability to Learn from Data
- 2. Module 2
- 1. Thinking in Machine Learning
- The human interface
- Design principles
- Types of questions
- Are you asking the right question?
- Tasks
- Classification
- Regression
- Clustering
- Dimensionality reduction
- Errors
- Optimization
- Linear programming
- Models
- Geometric models
- Probabilistic models
- Logical models
- Features
- Unified modeling language
- Class diagrams
- Object diagrams
- Activity diagrams
- State diagrams
- Summary
- 2. Tools and Techniques
- Python for machine learning
- IPython console
- Installing the SciPy stack
- NumPY
- Constructing and transforming arrays
- Mathematical operations
- Matplotlib
- Pandas
- SciPy
- Scikit-learn
- Summary
- 3. Turning Data into Information
- What is data?
- Big data
- Challenges of big data
- Data volume
- Data velocity
- Data variety
- Data models
- Data distributions
- Data from databases
- Data from the Web
- Data from natural language
- Data from images
- Data from application programming interfaces
- Challenges of big data
- Signals
- Data from sound
- Cleaning data
- Visualizing data
- Summary
- 4. Models Learning from Information
- Logical models
- Generality ordering
- Version space
- Coverage space
- PAC learning and computational complexity
- Tree models
- Purity
- Rule models
- The ordered list approach
- Set-based rule models
- Summary
- Logical models
- 5. Linear Models
- Introducing least squares
- Gradient descent
- The normal equation
- Logistic regression
- The Cost function for logistic regression
- Multiclass classification
- Regularization
- Summary
- Introducing least squares
- 6. Neural Networks
- Getting started with neural networks
- Logistic units
- Cost function
- Minimizing the cost function
- Implementing a neural network
- Gradient checking
- Other neural net architectures
- Summary
- 7. Features How Algorithms See the World
- Feature types
- Quantitative features
- Ordinal features
- Categorical features
- Operations and statistics
- Structured features
- Transforming features
- Discretization
- Normalization
- Calibration
- Principle component analysis
- Summary
- Feature types
- 8. Learning with Ensembles
- Ensemble types
- Bagging
- Random forests
- Extra trees
- Boosting
- Adaboost
- Gradient boosting
- Ensemble strategies
- Other methods
- Summary
- 9. Design Strategies and Case Studies
- Evaluating model performance
- Model selection
- Gridsearch
- Learning curves
- Real-world case studies
- Building a recommender system
- Content-based filtering
- Collaborative filtering
- Reviewing the case study
- Insect detection in greenhouses
- Reviewing the case study
- Building a recommender system
- Machine learning at a glance
- Summary
- 1. Thinking in Machine Learning
- 3. Module 3
- 1. Unsupervised Machine Learning
- Principal component analysis
- PCA a primer
- Employing PCA
- Introducing k-means clustering
- Clustering a primer
- Kick-starting clustering analysis
- Tuning your clustering configurations
- Self-organizing maps
- SOM a primer
- Employing SOM
- Further reading
- Summary
- Principal component analysis
- 2. Deep Belief Networks
- Neural networks a primer
- The composition of a neural network
- Network topologies
- Restricted Boltzmann Machine
- Introducing the RBM
- Topology
- Training
- Applications of the RBM
- Further applications of the RBM
- Introducing the RBM
- Deep belief networks
- Training a DBN
- Applying the DBN
- Validating the DBN
- Further reading
- Summary
- Neural networks a primer
- 3. Stacked Denoising Autoencoders
- Autoencoders
- Introducing the autoencoder
- Topology
- Training
- Denoising autoencoders
- Applying a dA
- Introducing the autoencoder
- Stacked Denoising Autoencoders
- Applying the SdA
- Assessing SdA performance
- Further reading
- Summary
- Autoencoders
- 4. Convolutional Neural Networks
- Introducing the CNN
- Understanding the convnet topology
- Understanding convolution layers
- Understanding pooling layers
- Training a convnet
- Putting it all together
- Applying a CNN
- Understanding the convnet topology
- Further Reading
- Summary
- Introducing the CNN
- 5. Semi-Supervised Learning
- Introduction
- Understanding semi-supervised learning
- Semi-supervised algorithms in action
- Self-training
- Implementing self-training
- Finessing your self-training implementation
- Improving the selection process
- Contrastive Pessimistic Likelihood Estimation
- Self-training
- Further reading
- Summary
- 6. Text Feature Engineering
- Introduction
- Text feature engineering
- Cleaning text data
- Text cleaning with BeautifulSoup
- Managing punctuation and tokenizing
- Tagging and categorising words
- Tagging with NLTK
- Sequential tagging
- Backoff tagging
- Creating features from text data
- Stemming
- Bagging and random forests
- Testing our prepared data
- Cleaning text data
- Further reading
- Summary
- 7. Feature Engineering Part II
- Introduction
- Creating a feature set
- Engineering features for ML applications
- Using rescaling techniques to improve the learnability of features
- Creating effective derived variables
- Reinterpreting non-numeric features
- Using feature selection techniques
- Performing feature selection
- Correlation
- LASSO
- Recursive Feature Elimination
- Genetic models
- Performing feature selection
- Engineering features for ML applications
- Feature engineering in practice
- Acquiring data via RESTful APIs
- Testing the performance of our model
- Twitter
- Translink Twitter
- Consumer comments
- The Bing Traffic API
- Deriving and selecting variables using feature engineering techniques
- The weather API
- Acquiring data via RESTful APIs
- Further reading
- Summary
- 8. Ensemble Methods
- Introducing ensembles
- Understanding averaging ensembles
- Using bagging algorithms
- Using random forests
- Applying boosting methods
- Using XGBoost
- Using stacking ensembles
- Applying ensembles in practice
- Understanding averaging ensembles
- Using models in dynamic applications
- Understanding model robustness
- Identifying modeling risk factors
- Strategies to managing model robustness
- Understanding model robustness
- Further reading
- Summary
- Introducing ensembles
- 9. Additional Python Machine Learning Tools
- Alternative development tools
- Introduction to Lasagne
- Getting to know Lasagne
- Introduction to TensorFlow
- Getting to know TensorFlow
- Using TensorFlow to iteratively improve our models
- Knowing when to use these libraries
- Introduction to Lasagne
- Further reading
- Summary
- Alternative development tools
- 10. Chapter Code Requirements
- 1. Unsupervised Machine Learning
- A. Biblography
- Index
- Назва: Python: Deeper Insights into Machine Learning. Deeper Insights into Machine Learning
- Автор: David Julian, Sebastian Raschka, John Hearty
- Оригінальна назва: Python: Deeper Insights into Machine Learning. Deeper Insights into Machine Learning
- ISBN: 9781787128576, 9781787128576
- Дата видання: 2016-08-31
- Формат: Eлектронна книга
- Ідентифікатор видання: e_2wr6
- Видавець: Packt Publishing